The emergence of Generative AI (GenAI) with Large Language Models (LLM) has unlocked promises of revolutionary possibilities driving newer business opportunities and customer experiences. It is not surprising that leading technology innovators like Microsoft, Google, Meta, NVIDIA, IBM, Databricks, Snowflake, and many others are investing heavily in LLMs to create business value for the next decade.
If GenAI delivers in line with the early signs then the scale of economic impact will be huge. McKinsey Global Institute estimates that generative AI will add between $2.6 and $4.4 trillion in annual value to the global economy, increasing the economic impact of Artificial Intelligence as a whole by 15% to 40%.
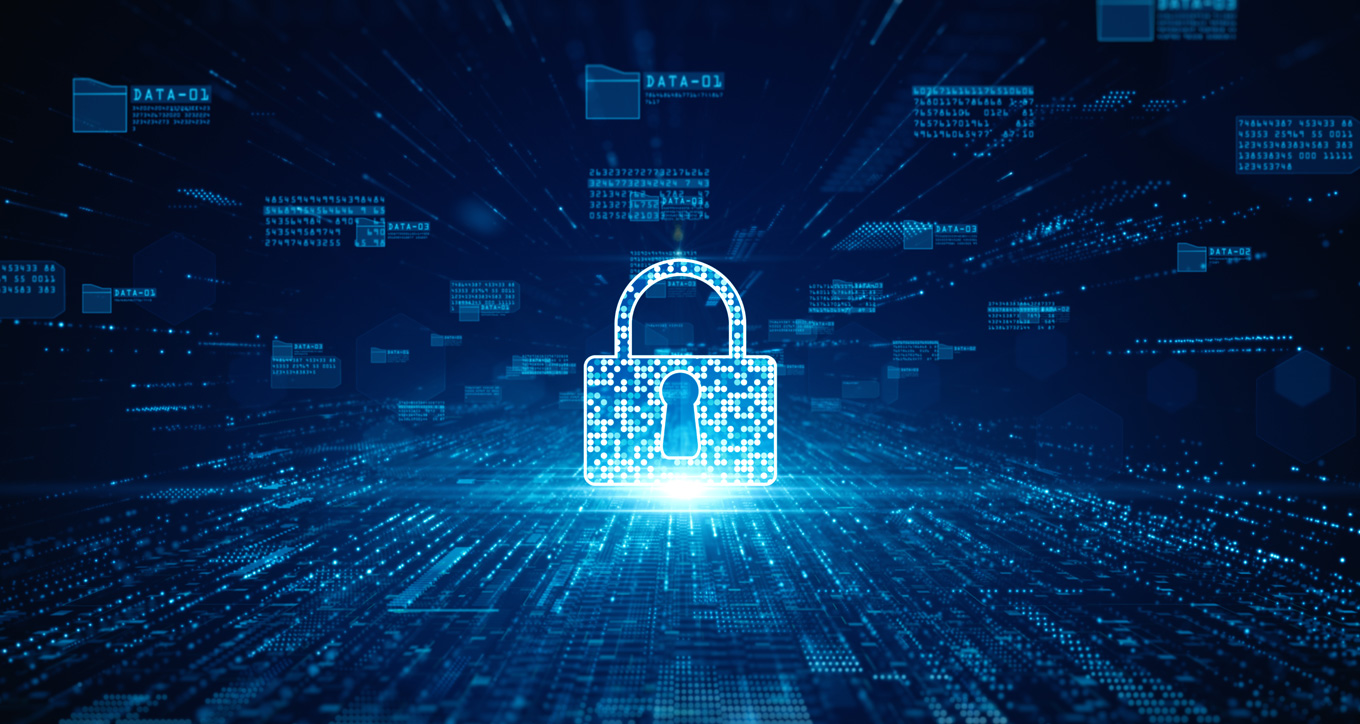
In this article, I will focus on the implications of GenAI on data security, privacy, and governance, and discuss how organizations can adapt to ensure a secure and responsible future.
Implications of GenAI on data security
Data is critical to every business and it makes each enterprise unique and different from others. As per a study, one of the largest multinational oil and gas companies, for predictive maintenance alone, the company has 17,000 models and 4 trillion rows of data — adding another 20 billion per day from 3 million sensors across facilities around the world.
Every industry will be immensely disrupted by leveraging GenAI’s breakthrough capability; content generation, personalization, and data augmentation driving innovation in numerous areas by generating diverse and sophisticated data.
However, with great power comes great responsibility, and the advent of GenAI raises significant concerns for data security, privacy, and governance. These newer realms will affect organizations for managing their internal as well as customer and partner data, which has a serious impact on businesses if compromised. Keeping this in mind, it is wise to prepare for this future and navigate the complexities that come with it.
New Frontiers: Data Security Challenges
- Commercial IP and Privacy protection: One of the grave dangers for IP-driven business strategies may come from the reckless use of reams of confidential data to train the LLMs and GenAI, which may lead to copyright infringement, privacy, and security breaches. That will open up a pandora’s box and bad actors would be keen to lay their hands on internal documentation, data, and strategies through unidentified and unattended loopholes.
- Synthetic Data Vulnerabilities: The generation of realistic synthetic data by GenAI can enhance machine learning models but also opens avenues for malicious actors to create convincing fake data, leading to potential misinformation and data poisoning.
- Integrity and Authenticity of LLM Models: LLMs are intelligently trained models from vast troves of data but they do not differentiate true from false, which impacts the bias of their content generation. Hence the possibility of generating convincing fake data, such as deep fakes, poses risks to data integrity and authenticity. This could lead to misinformation or fraudulent activities.
- Data Privacy and Anonymization: Traditional data anonymization techniques may not suffice to protect individual privacy when faced with the power of GenAI in reidentifying anonymized data.
- Ownership and Consent: The use of GenAI to generate content may blur the lines of data ownership and consent. Organizations must devise, clarify and establish data ownership and obtain explicit user consent when dealing with data generated by GenAI systems.
- Biometric Data Privacy: GenAI’s ability to create realistic images raises concerns about biometric data privacy. Biometric data, such as facial images, could be generated and used without individuals’ consent, potentially leading to privacy violations.
- Transparency: The inherent complexity of GenAI models demands increased transparency to build trust with users, regulators, and stakeholders. Understanding how AI-generated content is produced becomes critical for responsible deployment.
- Regulatory Compliance: Incorporating GenAI in data processing and decision-making may raise regulatory compliance challenges. Organizations must ensure that their use of GenAI aligns with data protection regulations and industry standards.
- Adversarial Attacks: GenAI models can be susceptible to adversarial attacks, where manipulated inputs cause AI systems to produce inaccurate results. Ensuring model accuracy and security against such attacks becomes crucial.
- AI-driven Cyber Threats: As GenAI advances, cybercriminals may exploit AI-driven attack vectors, making it essential for organizations to develop AI-powered cybersecurity measures to detect and mitigate AI-based threats.
Securing Data Horizons in the Era of GenAI
But the question is what should enterprises embrace to address the data security challenges in the era of GenAI. Here, I am sharing some of the key strategies that enterprises should evaluate, embrace and adapt. It’s nothing in the space of data security but it needs a more refined framework to implement.
- Futuristic Data infrastructure to manage the exponentially growing data: As we prepare to adapt to the GenAI wave, enterprises must manage the exponentially growing data with a unified and consistent governance approach. Unless we set up the right data management capabilities (data warehouse/ lake house, quality, catalog, and lineage), it creates foundational challenges even before we prepare for data security.
- Data-Centric Security Approach: A data-centric security strategy, focused on protecting the data itself throughout its lifecycle, becomes paramount in the age of GenAI.
- Robust Data Security Measures: Organizations must invest in state-of-the-art security solutions, including AI-powered threat detection and incident response systems.
- Responsible AI Governance: Implementing governance frameworks that prioritize ethical use, fairness, and accountability of AI models is essential to ensure responsible AI deployment.
- Building Culture around Data Security and Privacy: Culture plays a big role in ensuring the implementation of data security practices, processes, and technologies is successful. Regular training and awareness programs educating them about data security & privacy best practices, potential threats, and the importance and impact of privacy compliance must be implemented and the efficacy should be measured regularly.
Conclusion
The age of GenAI brings unparalleled potential for progress, but it also demands a profound commitment to data security, privacy, and governance. As we step into the age of GenAI, data executives and data security professionals are encouraged to embrace responsible data-centric security strategies, prioritize transparency and accountability, and foster an ethical AI culture.
By navigating the complexities of GenAI with foresight and diligence, businesses can unlock the transformative power of AI while upholding the values of data privacy, security, and responsible innovation.
Together, let us shape a future where GenAI serves as a catalyst for positive change and empowers organizations to thrive in a secure digital landscape.
The same blog is posted on my Medium.com site as well. Please refer here.
I like your post Shubo and especially how crisp and to the point texts you have mentioned. I am also in Microsoft Database technologies, and I think developers like me have to see and adapt such technology shifts very soon. :)
ReplyDelete